From plain text to AI’s generate video
WHY THIS MATTERS IN BRIEF
In recent years, “synthetic media” has become a general term used to describe video, image, text, and voice that computers generate. With these advances, we are about to see a major paradigm shift in media creation. Companies like Rosebud AI and Humen are disrupting the multimedia creation space by synthesizing videos and images, potentially saving creative agencies and studios millions of dollars in asset creation. Imagine not being able to program or code, but still being able to write a description or a script and have an AI create an HD image or video of it for you, this is the technology that’s now arriving.
In 2016 an Artificial Intelligence (AI) won an award for best short film at the Cannes Film Festival in France, in 2017 another created the world’s first AI music album for Sony, and elsewhere others began innovating and creating everything from winter scenes to help create better self-driving cars, to new product designs, including clothing, sneakers and even the world’s first self-evolving robot. And all these AI’s have one thing in common – they’re all “creative.”
AI is getting better and better at creating what’s known as “Generative content,” in short, content, such as images, music and scripts, or, let’s face it, text, that AI’s are able to make by themselves with little or, as is more the case, no input from humans, and recent examples include photo-realistic images of fake celebrities and an increasing number of new, other, AI composed music albums from artists such as Amper, DeepBach, Magenta, and Flow Machines, all AI’s. Now though scientists are working on building AI’s that can create generative video. The idea is that simply by typing out a phrase AI could create a video of that scene, and scientists at Duke University and Princeton University, following on from Microsoft who recently unveiled their own version that does the same but just for images, have created a working model.
“Video generation is intimately related to video prediction,” say the authors in their new paper. Video prediction, where AI attempts to predict what actions come next in a video, has long been a goal of many AI researchers, and for obvious reasons, security companies, but so far, other than a product preview from MIT whose AI managed to predict what happened next in a cycle race, there have been relatively few successes.
Visual representations, however, especially moving ones, often contain a wide variety actions and outcomes so as a first step the researchers used a narrow range of easily defined activities, which they took from Google’s Kinetics Human Action Video Dataset, for their AI to learn from including sports, such as cycling, football, golf, hockey, jogging, sailing, swimming and water skiing. The AI then studied these clips and learnt to identify each motion, refining its neural network and refining itself all the time.
With a dataset in place, the researchers then used a two step process to create the generative video. The first step was to create an AI that could generate video based on just a text description, and then came the second stage, the creation of a second “Discriminator” AI.
For example, if the text input was to create a video of “biking in snow,” the first AI would produce a video and the second, the discriminator would judge it and compare it to a real video of someone biking in the snow, and any improvements or recommendations would be automatically fed back into the model so that over time the results got better and better until the generative video was indistinguishable from the real thing.
While the teams work is still in its earliest stages, with the new AI only capable of creating videos that are 32 frames long and the size of a postage stamp, over time they will get longer, bigger and better quality, and as it turns out the AI is finding humans, with our bodies and our unpredictable actions, the most problems, but to get a better grasp on us flesh bags the team are now training it to understand how the human skeleton works.
Beyond the obvious nightmare of fake news generation, an example of which I showed off recently during my talk on the Future of Trust in London, where another generative AI was used to create a thoroughly convincing fake Obama news clip, there could be actual use for generative video, such as using it to help train self-driving cars better by helping produce realistic road and traffic simulations, or helping athletes train better by simulating game play.
Either way it’ll be a while before we see any AI produced films, but we’re now at the start of our journey, and if following AI developments has taught me one thing, it won’t be decades before we see one, it’ll be years.
線上諮詢
與我們合作,馬上展開全新的創作里程碑
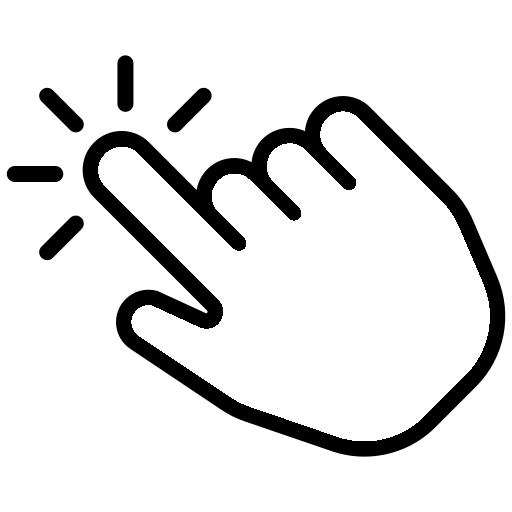
- 📅 立即預約,30 秒完成!
- 🎯 與創辦人 1 對 1 交流,獲得專屬建議! 🎯 與創辦人 1 對 1 交流!